Is It MCAR Or Little's MCAR? Unveiling The Mystery Behind Missing Data Mechanisms
Missing data can be a real headache for researchers and analysts alike. If you're diving into the world of data science or statistics, you've probably come across terms like MCAR and Little's MCAR. But what's the difference? Is it MCAR or Little's MCAR that you should be focusing on? Let's break it down in a way that's easy to digest, while still being super informative.
Picture this: you're working on a massive dataset, ready to uncover groundbreaking insights, but then BAM! You hit a wall of missing values. Now, before you panic, it's important to understand why those values are missing. This isn't just about filling in blanks; it's about understanding the underlying mechanisms that caused the data to go MIA.
In this article, we'll dive deep into the world of missing data, focusing on the two big players: MCAR (Missing Completely at Random) and Little's MCAR test. We'll explore what they mean, how they differ, and why they matter. By the end, you'll have a solid grasp of which one to use and when. So, buckle up and let's get started!
Read also:How Old Is Luciacutea Meacutendez The Age Biography And Fascinating Journey Of A Mexican Icon
Understanding Missing Data Mechanisms
Before we jump into MCAR vs Little's MCAR, let's first understand the basics of missing data mechanisms. There are three main types: Missing Completely at Random (MCAR), Missing at Random (MAR), and Missing Not at Random (MNAR). Each type has its own implications for data analysis, and understanding them is crucial for accurate results.
What is MCAR?
MCAR, or Missing Completely at Random, is the simplest and most desirable type of missing data mechanism. When data is MCAR, the missingness is completely random and unrelated to any observed or unobserved variables. Think of it like flipping a coin – there's no pattern to predict which values will be missing.
Here are some key characteristics of MCAR:
- The probability of missing data is the same for all cases.
- There's no relationship between the missingness and the variables in your dataset.
- MCAR is often considered the "best-case scenario" for missing data because it minimizes bias in your analysis.
Little's MCAR Test: What Is It?
Now, here's where things get interesting. While MCAR sounds great in theory, how do you actually determine if your data is truly MCAR? Enter Little's MCAR test. This statistical test helps you assess whether your missing data can be considered MCAR.
How Does Little's MCAR Test Work?
Little's MCAR test uses a multivariate test of means to compare the means of observed and missing data across all variables. If the test shows no significant differences, it suggests that the data is MCAR. However, if there are significant differences, it indicates that the data might not be MCAR.
Here's a quick rundown of the process:
Read also:Ark Brewpub The Ultimate Craft Beer Experience Youve Been Missing
- Perform a chi-square test to compare means across observed and missing data.
- Check the p-value – if it's greater than 0.05, you can assume MCAR.
- Remember, though, that Little's test has limitations and should be used in conjunction with other analyses.
MCAR vs Little's MCAR: What's the Difference?
So, what's the real difference between MCAR and Little's MCAR? Well, MCAR is a theoretical concept that describes how missing data behaves, while Little's MCAR test is a practical tool to evaluate whether your data fits this description. Think of MCAR as the idea and Little's test as the method to test that idea.
When Should You Use Little's MCAR Test?
Little's MCAR test is particularly useful when you need to validate whether your missing data can be treated as MCAR. However, it's not a one-size-fits-all solution. Here are some scenarios where Little's test shines:
- When you have a large dataset with multiple variables.
- When you suspect that your missing data might be random but want to confirm it statistically.
- When you're working with sensitive data where bias could have serious consequences.
Why Does MCAR Matter?
MCAR matters because it directly impacts the validity of your analysis. If your data is truly MCAR, you can use simpler imputation methods without worrying about introducing bias. On the other hand, if your data isn't MCAR, you'll need to explore more complex techniques to ensure accurate results.
Real-World Implications of MCAR
Let's look at a real-world example. Imagine you're conducting a survey about income levels in a particular region. If some respondents fail to provide their income data, but their missingness is unrelated to any other variables (like age, gender, or education), then the data is likely MCAR. This means you can confidently use methods like mean imputation without skewing your results.
Common Misconceptions About MCAR
There are a few common misconceptions about MCAR that can trip up even the most seasoned analysts. Here are a few to watch out for:
- MCAR means all missing data is random: Not necessarily. Just because some data is MCAR doesn't mean all missing data in your dataset is random.
- Little's test is foolproof: While it's a powerful tool, Little's test isn't perfect. It assumes multivariate normality and can sometimes produce misleading results.
- MCAR is always the best option: While MCAR is ideal, it's not always achievable. Sometimes, MAR or even MNAR might be more appropriate depending on your dataset.
Best Practices for Handling Missing Data
Now that you understand the difference between MCAR and Little's MCAR, let's talk about best practices for handling missing data. Here are a few tips to keep in mind:
- Always start by identifying the missing data mechanism (MCAR, MAR, or MNAR).
- Use Little's MCAR test to validate your assumptions about missingness.
- Choose the right imputation method based on your data's characteristics.
- Document your process thoroughly to ensure transparency and reproducibility.
Advanced Techniques for Missing Data
If your data isn't MCAR, don't worry – there are plenty of advanced techniques to handle missing values. Some popular methods include:
- Multiple Imputation: Creates multiple plausible values for missing data based on observed data.
- Maximum Likelihood Estimation: Uses all available data to estimate parameters without imputation.
- Pattern Mixture Models: Accounts for different missing data patterns in your analysis.
Case Studies: MCAR in Action
Let's take a look at a couple of case studies where MCAR played a crucial role in data analysis.
Case Study 1: Healthcare Data
In a study examining patient recovery rates, researchers found that some patients failed to complete follow-up surveys. Using Little's MCAR test, they determined that the missingness was random and unrelated to patient outcomes. This allowed them to use simpler imputation methods without compromising the study's validity.
Case Study 2: Education Data
A team analyzing standardized test scores discovered missing values in their dataset. After conducting Little's MCAR test, they found that the missingness was not random. This led them to explore more complex methods like multiple imputation, ensuring that their results were accurate and unbiased.
Resources and Tools for Handling Missing Data
There are plenty of resources and tools available to help you handle missing data effectively. Here are a few to consider:
- R and Python Libraries: Packages like 'mice' in R and 'fancyimpute' in Python offer powerful tools for imputation and missing data analysis.
- Statistical Software: Programs like SPSS and SAS provide built-in functions for handling missing data.
- Online Tutorials: Websites like Coursera and Khan Academy offer courses on missing data mechanisms and imputation techniques.
Conclusion: Is It MCAR or Little's MCAR?
In conclusion, understanding whether your data is MCAR or requires Little's MCAR test is essential for accurate analysis. By identifying the missing data mechanism and using the right tools, you can ensure that your results are reliable and unbiased.
So, what's next? If you've found this article helpful, why not share it with your colleagues or leave a comment with your thoughts? And don't forget to check out our other articles for more insights into the world of data science and statistics.
Daftar Isi
- Understanding Missing Data Mechanisms
- What is MCAR?
- Little's MCAR Test: What Is It?
- MCAR vs Little's MCAR: What's the Difference?
- Why Does MCAR Matter?
- Common Misconceptions About MCAR
- Best Practices for Handling Missing Data
- Case Studies: MCAR in Action
- Resources and Tools for Handling Missing Data
- Conclusion: Is It MCAR or Little's MCAR?

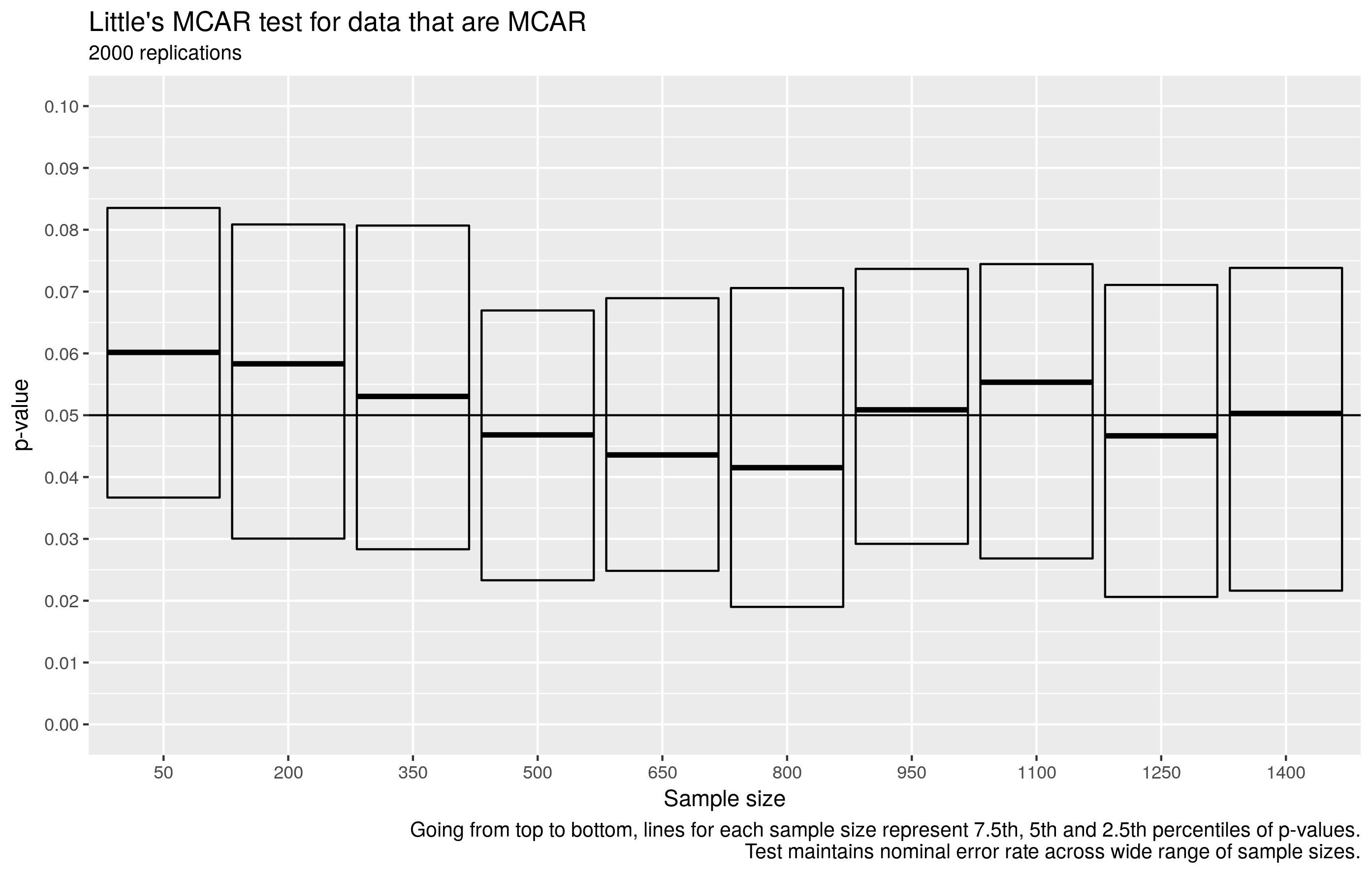
